Ahmad Khalifa
Artificial Intelligence (AI) is a transformative field that leverages input data processed through predefined algorithms within an AI system to produce specific, problem-solving outputs. It encompasses a wide range of techniques and technologies, making it a powerful tool for addressing complex tasks and challenges, especially in the field of Ophthalmology which heavily relies on complex patterns and signs of disease. In simple terms, AI is the process of feeding a system with inputs and desired outputs so it can learn to make predictions or decisions based on patterns in the data. Thus, providing problem-solving solutions guided by an algorithm. Usually, the instructions (algorithm) need to be in a language that the machine can interpret to solve problems (Figure 1).
This article will discuss where we currently stand in the use of retinal Imaging methods with the aid of AI for the development of ophthalmological practice. A specific implementation of AI that is available now relies on pattern recognition and grouping of images with similar findings and patterns which can be used to aid in the research and development of a better understanding of retinal pathologies, particularly chronic ones. However, the prospective use that I want to discuss is the clinical aid of AI in early triage for use by non-ophthalmology clinicians who usually have not one but multiple barriers in providing enough information for an effective referral/handover to more senior clinicians specializing in Ophthalmology.
Moreover, the essay will discuss the expansion in use for detecting emergency pathologies as a first tool in managing visual complaints in the emergency department or on the wards made by inpatients.
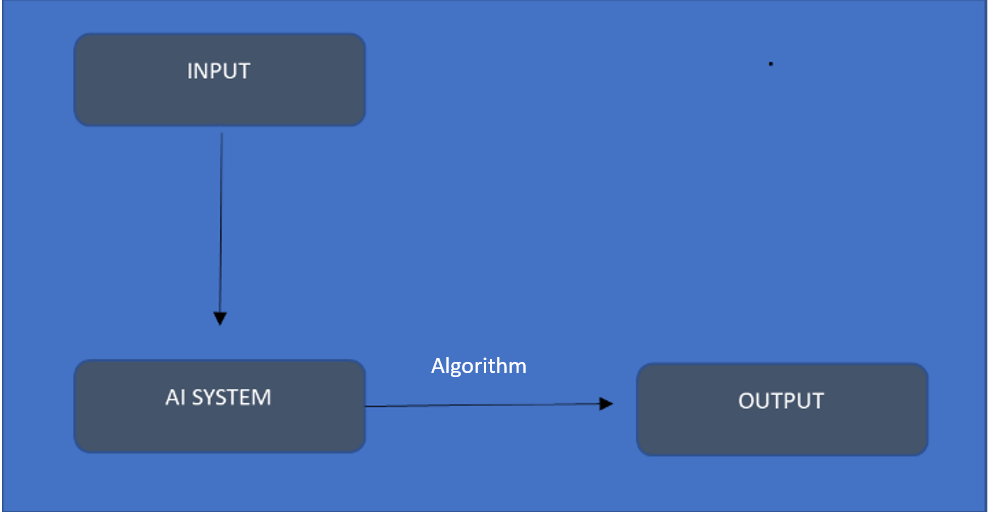
(Figure 1: Simplified schematic of data flow in basic artificial intelligence software)
Artificial intelligence (AI) has been an emerging tool that professionals from different sectors use to make processes less challenging and provide a cost-effective tool for quick problem-solving. Particularly in the field of Ophthalmology, it could be used for early diagnosis, providing a better outcome for patients, especially for sight preservation.
Currently, AI is used in imaging techniques and reporting systems in Ophthalmology software, which provides accurate data measurements that would otherwise be difficult and time-consuming for doctors (1). AI can process clinical imaging and provide a suggestion based on the guidelines fed into the algorithm. Its current use has been extensive, especially for retinal imaging. Retinal pathologies such as diabetic retinopathy (DR) that is a major complication of diabetes (2). The burden of diabetes is continuously increasing and the number of people with the condition is estimated to be 600 million by 2040 (3). DR is classified in stages; early stages are reversible if detected early alongside sufficient appropriate glycemic control (4). Each stage comes with different hallmarks for identifying the stage and essentially providing sufficient treatment improving patient outcomes. Furthermore, AI can be implemented widely in underserved areas where there is a lack of accessibility to healthcare and professionals of a certain standard. A study was done in India to provide an outlook on the effectiveness as well as the financial perks of using AI for screening for diabetic retinopathy. This aimed to lift off some of the load on the Ophthalmology departments (5,6). This will lift the burden off clinicians, providing a ready set of diagnostic findings that can be monitored by a clinician to take on the suggestion made by the AI-run system. The observational study found that the performance of the automated system in detecting moderate or worse diabetic retinopathy was equal to or exceeded manual grading. The study has shown that there is a prospective feasibility of making these screening programmes national or even international especially in under-served areas. Therefore, having such services can be beneficial as they can be monitored and audited using telemedicine programmes that can transmit data across sites or even continents for professionals to review and monitor the overall performance of the programmes (7).
However, such programmes would not be all automatically run and would need input from professionals of different backgrounds, some in health and others in technology, which is not fully advocated for currently. A final criticism of the screening programme is that it struggles to explore the need for trained professionals specialising in running such systems with a good background in healthcare. Although it provides good outcomes as the study only looks at two medical centres, there are surely more challenges to overcome when the programme is extrapolated to fit a bigger population of patients.
Thus, the need for trainees of such calibre to take on these roles is needed, which is still limited as there isn’t an official post for training that combines technology and ophthalmology. The Standing Committee of European Doctors (CPME) suggested the need to implement AI systems in basic and continuing medical education. To provide basic training as part of the residency programmes and the basics of how AI works. As far as medical education is concerned, developing curriculum proposals specifically designed to train future physicians on AI is needed, if not now but in the very near future (8).
The AI movement needs to be discussed in greater depth considering the need for an ethical and professional regulating body, in order to maintain accuracy and best outcomes at the point of service.
Using AI for aid in service provision needs to be looked at from multiple perspectives, including the user and the patients. A few questions need to be addressed. What happens to the data that is being collected? How does it affect the algorithm development and essentially the output and in the overall picture the specificity of future diagnostics that are made based on the outputs provided by the system? How do patients feel knowing that a major part of their management plan is being inputted by a computer? Are they happy to allow for their clinical pictures to be part of the algorithm?
These are questions that need to be addressed in terms of patient autonomy, the choice of the treating body and finally privacy. In terms of users, there has always been that question that has been roaming around since the start of the technology wave. Will AI replace doctors for diagnosing purposes, and how will it affect training and essentially the competency of newly qualified Ophthalmologists? Relying on AI for diagnosing can take the stimulating part of clinical practice that is used as part of case-based learning, in which a case is discussed by trainees with their mentors to provide different realms of experience and essentially better learning opportunities, to aid in making more holistic practitioners.
Secondly, how can we use artificial intelligence (AI) in clinical practice when faced with patients with visual issues as newly qualified doctors? Comparing the more traditionally taught methods such as direct Ophthalmoscopy and how there are many barriers to it (9). As well as the fact it is becoming less useful outside Ophthalmology clinics in picking up signs for emergency presentations which can lead to blindness (10). New-starting doctors struggle to make effective referrals to specialized units such as the Ophthalmology team, when presented with visual problems on the wards or in the emergency department. They usually call for help with minimal key information to hand over or without even examining the back of the eyes. This burdens the Ophthalmology team and often causes unnecessary referrals to be dealt with.
The suggestion is to provide software that can extract pictures from smartphones with an ophthalmoscope-like extension attached to the camera of the phone (11). Followed by storing them in the AI software that processes them, providing a standardized interpretation of the retina that could be used for a more useful handover. However, this would be easier to accomplish and integrate into practice if AI was to be added to the medical education curriculum. The need to clearly find an educational role for artificial intelligence still exists and will need to be thoroughly examined and reviewed. As it will help bridge the gap that trainees struggle with in getting enough time for professional development and mentorship during training. This will find an effective application in the trainee’s roles to aid in early practicing years to provide safer care for patients and a more informative day-to-day practice. This method would overall be educational and helpful in saving time to see more patients effectively and referring those who need input from more senior Ophthalmologists. Realistically, they have very little time to spend with every patient due to the high burden of visual problems the communities suffer from.
Overall, AI has many applications in Ophthalmology, but the need for prompt review and implementation is needed to provide a more complete practice that could be used in all environments. A particularly influential wider application of AI, alongside an effective telemedical system, with the aid of professionals from abroad could provide greater health equality around the world, especially in low socioeconomic areas. This would help focus funds on greater health and educational needs of those areas therefore improving the overall quality of life and providing a more prosperous future for the new generations to come.
References
- Xie, Y. et al. (2020) ‘Artificial Intelligence for teleophthalmology-based diabetic retinopathy screening in a national programme: An economic analysis modelling study’, The Lancet Digital Health, 2(5). doi:10.1016/s2589-7500(20)30060-1.
- Daniel Shu Wei Ting, M. (2017) Machine learning screen for diabetic retinopathy and other eye diseases, JAMA. Available at: https://jamanetwork.com/journals/jama/article-abstract/2665775 (Accessed: 03 September 2023).
- Yau, J.W.Y. et al. (2012) Global prevalence and major risk factors of diabetic retinopathy, American Diabetes Association. Available at: https://diabetesjournals.org/care/article/35/3/556/28568/Global-Prevalence-and-Major-Risk-Factors-of (Accessed: 03 September 2023).
- Shukla, U. and Tripathy, K. (no date) Diabetic retinopathy – statpearls – NCBI bookshelf, Diabetic retinopathy. Available at: https://www.ncbi.nlm.nih.gov/books/NBK560805/ (Accessed: 03 September 2023).
- Gulshan, V. (2019) Performance of a deep-learning algorithm vs manual grading for detecting diabetic retinopathy in India, JAMA Ophthalmology. Available at: https://jamanetwork.com/journals/jamaophthalmology/article-abstract/2734990 (Accessed: 03 September 2023).
- Author links open overlay panel Sudhir Rachapelle MD et al. (2012) The cost–utility of telemedicine to screen for diabetic retinopathy in India, Ophthalmology. Available at: https://www.sciencedirect.com/science/article/abs/pii/S0161642012008615 (Accessed: 04 September 2023).
- Xie, Y. et al. (2020) ‘Artificial Intelligence for teleophthalmology-based diabetic retinopathy screening in a national programme: An economic analysis modelling study’, The Lancet Digital Health, 2(5). doi:10.1016/s2589-7500(20)30060-1.
- Civaner, M.M. et al. (2022) Artificial Intelligence in medical education: A cross-sectional needs assessment, BMC Medical Education. Available at: https://www.ncbi.nlm.nih.gov/pmc/articles/PMC9646274/ (Accessed: 04 September 2023).
- Mackay DD; Garza PS; Bruce BB; Newman NJ; Biousse V, D.D. (no date) The demise of direct ophthalmoscopy: A modern clinical challenge, Neurology. Clinical Practice. Available at: https://pubmed.ncbi.nlm.nih.gov/26137422/ (Accessed: 04 September 2023).
- AM, K. (no date) Direct ophthalmoscopy in the evaluation of emergency department patients with headache: Time to rethink?, Emergency Medicine Australasia: EMA. Available at: https://pubmed.ncbi.nlm.nih.gov/36628956/ (Accessed: 04 September 2023).
- Mamtora, S. et al. (2018) Smart phone ophthalmoscopy: A potential replacement for the direct ophthalmoscope, Nature News. Available at: https://www.nature.com/articles/s41433-018-0177-1 (Accessed: 04 September 2023).